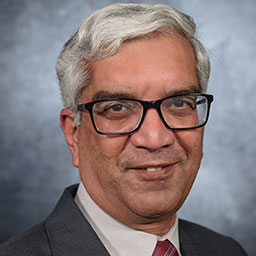
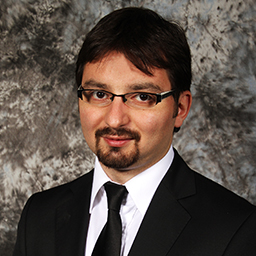
A paper co-authored by two Naveen Jindal School of Management faculty members and an Erik Jonsson School of Engineering and Computer Science PhD graduate has won a best paper award for research that helps reduce bias in medical diagnoses.
Dr. Srinivasan Raghunathan, an Ashbel Smith Professor in Information Systems; Dr. Mehmet Ayvaci, an associate professor in Information Systems; and Dr. Mehmet Eren Ahsen, a 2015 biomedical engineering doctoral graduate now an assistant professor at the University of Illinois at Urbana-Champaign, won the award from Information Systems Research, a peer-reviewed academic journal that is tracked in The UTD Top 100 Business School Research Rankings™.
Their paper, “When Algorithmic Predictions Use Human-Generated Data: A Bias-Aware Classification Algorithm for Breast Cancer Diagnosis,” details a debiasing mechanism that the team developed to address algorithmic bias in the context of developing a decision-support tool for breast-cancer diagnosis.
Raghunathan became interested in this type of research about 10 years ago when he had asked a relative who is a radiologist why family histories are needed to interpret X-rays or mammograms.
“Not everything is black and white in an X-ray or a mammogram,” he said. “When radiologists are uncertain, when the picture is not conclusive, they use this information to come up with a better interpretation of whatever they see.”
Raghunathan began doing research into whether information such as family history can subjectively influence results during a process that is supposed to be objective.
Ayvaci, who joined the Jindal School faculty not long after Raghunathan began asking these questions, had done this type of research. They began collaborating soon thereafter.
“If the radiologist is uncertain about whether an assessment is positive or negative, and then you provide them additional information such as a family history of cancer, then the radiologist is more likely to assess that X-ray or mammogram finding as positive,” Raghunathan said. “That’s clearly an anchoring bias. They come up with this new information, and then they anchor on that.”
With the increased use of algorithms and machine-learning models in healthcare, Raghunathan and Ayvaci began exploring ways to mitigate the bias potential in computer-based decision-making processes, which use hundreds of thousands of input data, including potentially biased findings from radiologists.
“Data is something that we generate as human beings, and we have certain types of biases,” Ayvaci said. “Those biases become part of the data. When we feed that data into algorithms, they’re going to make similar errors as we do. The question is can we develop a mechanism that can address that kind of bias? Can we develop an algorithm to adjust for biases that exist in data sets?”
The problem as it relates to healthcare, Ayvaci said, is that these biases automate and even magnify problems that exist in the healthcare system.
“In healthcare, there’s a lot of value potential from algorithms,” he said. “Even though data is at the core of the delivery, at the same time we have to admit that there are going to be some vulnerabilities that we have to face. One of those is bias.”
As machine learning, which utilizes algorithms, becomes more pervasive in healthcare, Raghunathan said, accounting for bias helps the industry better realize that potential.
“Eliminating bias from the human side can be very, very challenging,” he said. “People have been trying that for many years. At a minimum, the algorithms now can recognize that bias, if it exists, and the machines can adjust to account for it.”